Alexander Mehta, William Yang
Independent, University of Pennsylvania
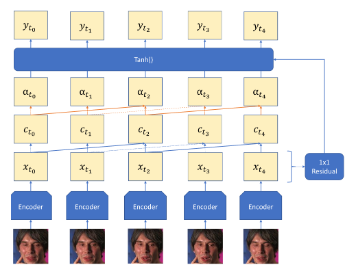
Abstract
In the task of emotion recognition from videos, a key improvement has been to focus on emotions over time rather than a single frame. There are many architectures to address this task such as GRUs, LSTMs, Self-Attention, Transformers, and Temporal Convolutional Networks (TCNs). How ever, these methods suffer from high memory usage, large amounts of operations, or poor gradients. We propose a method known as Neighborhood Attention with Convolutions TCN (NAC-TCN) which incorporates the benefits of attention and Temporal Convolutional Networks while ensuring that causal relationships are understood which results in a reduction in computation and memory cost. We accomplish this by introducing a causal version of Dilated Neighborhood Attention while incorporating it with convolutions. Our model achieves comparable, better, or state-of-the-art performance over TCNs, TCAN, LSTMs, and GRUs while requiring fewer parameters on standard emotion recognition datasets
BibTeX
@misc{mehta2023nactcn, title={NAC-TCN: Temporal Convolutional Networks with Causal Dilated Neighborhood Attention for Emotion Understanding}, author={Alexander Mehta and William Yang}, year={2023}, eprint={2312.07507}, archivePrefix={arXiv}, primaryClass={cs.CV} }